Go Back
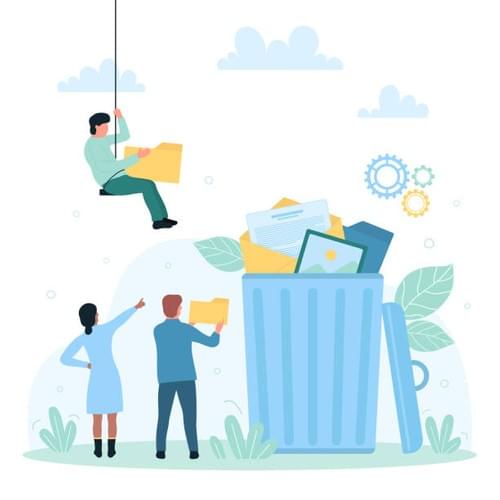
Cleaning and Preparation
The goal is to enhance data quality, facilitate meaningful analysis, and support the development of robust models for informed decision-making.
More Details
Data cleaning and preparation involve the process of identifying and rectifying errors, inconsistencies, and inaccuracies in raw datasets to ensure they are accurate, reliable, and ready for analysis. This crucial step in the data science pipeline includes tasks such as handling missing values, standardizing formats, removing duplicates, and addressing outliers. The goal is to enhance data quality, facilitate meaningful analysis, and support the development of robust models for informed decision-making.